Unleashing AI: Revolutionizing Pharmaceutical Systems and Clinical Data Management
- Nabil Attia
- May 31, 2023
- 5 min read
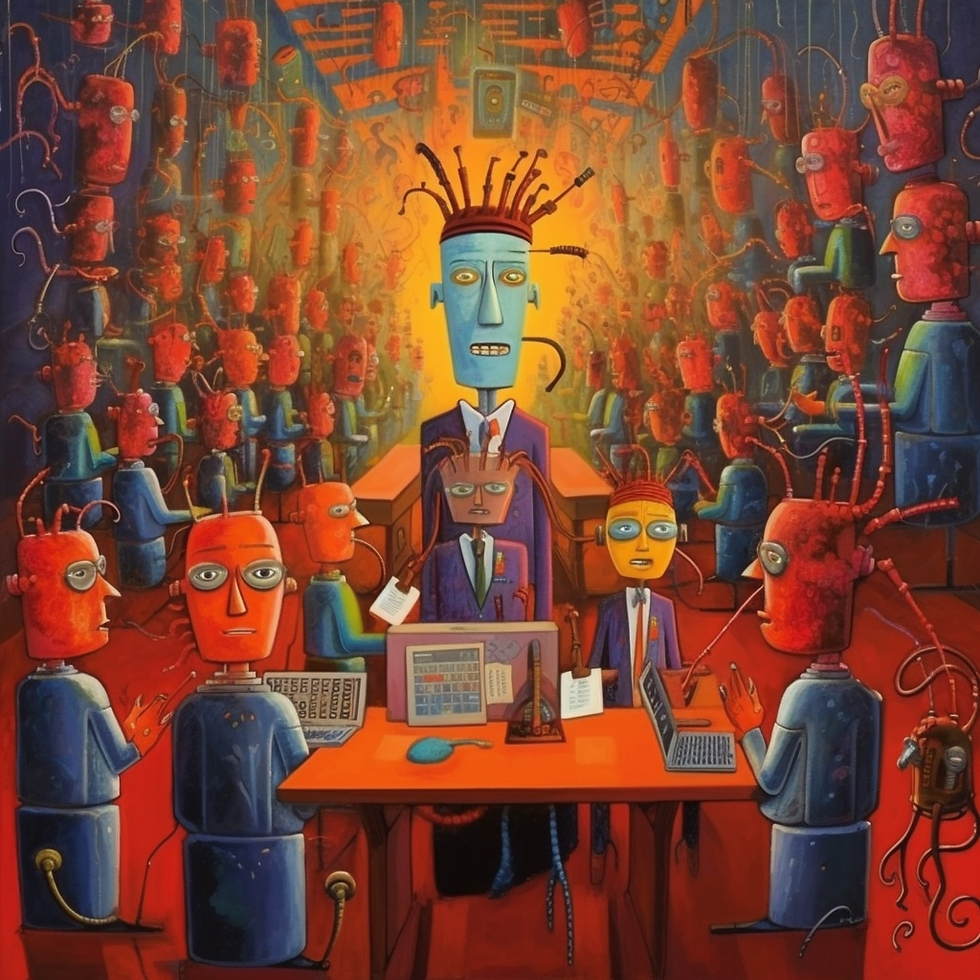
Artificial Intelligence (AI) and Machine Learning (ML) are rewriting the rules of many industries worldwide, and the pharmaceutical domain is part of this transformative journey. AI's impact is impressive, accelerating drug development, enhancing patient outcomes, increasing efficiency, and driving down costs. Among these, the role of AI in Clinical Data Management Systems (CDMS) stands as an extraordinary example of its transformative power.
1. The Expansive Influence of AI in Pharmaceutical Systems
AI's integration into the pharmaceutical sector is serving as a pivotal catalyst in areas such as drug discovery. By leveraging machine learning algorithms, AI can swiftly process vast amounts of data, generate new hypotheses, and predict the potential success of novel drugs, thereby accelerating their development and saving substantial resources.
Furthermore, AI employs tools like natural language processing (NLP) to extract critical information from a wealth of scientific literature and employs molecular modeling to design innovative molecules. These capabilities, coupled with machine learning's ability to identify patterns and correlations in biological data, enable pharmaceutical enterprises to forecast trends using predictive analytics. This empowers them to refine their strategies and streamline their operations.
Beyond its transformative role in pharmaceutical systems, AI is also driving a paradigm shift in the broader healthcare industry. It is enhancing patient care through AI-powered chatbots, which offer round-the-clock assistance, and diagnostic tools, which provide early and precise disease detection. AI's role extends into the operating room, where surgical robots deliver unparalleled precision.
The cost-saving benefits of AI in healthcare are equally notable. AI’s ability to automate administrative tasks leads to reductions in labor costs and errors. Moreover, AI's acceleration of drug discovery in the pharmaceutical sector not only expedites development but also significantly cuts down associated costs. The rise of AI-driven medical devices allows for remote patient monitoring, curbing hospital readmissions and reducing the expenses of traditional in-person visits.
Moreover, AI is a force multiplier in medical research. By sifting through and analyzing vast datasets, it aids in the creation of disease progression models and the design of efficient clinical trials, driving forward scientific discoveries and improving patient outcomes.
AI is also reshaping the healthcare landscape by enabling telemedicine, granting patients increased access to health information and care. It is fostering an environment of collaboration among healthcare providers, patients, and researchers, cultivating a more cohesive healthcare ecosystem.
Looking forward, AI heralds a new era of innovation in healthcare. It's poised to introduce personalized medicine and precision healthcare, fast-track medical research and innovation, and address important ethical challenges, such as data privacy and algorithmic bias. However, to unlock AI's full potential in healthcare, robust governance frameworks must be put in place and investments in AI education and training need to be made, ensuring patient safety and privacy.
2. AI: The Catalyst in Clinical Data Management Systems

Clinical trials, a vital facet of drug development, have historically been resource-intensive and expensive. The advent of AI, however, is changing this narrative, enhancing efficiency and reducing costs in multiple ways:
- Enhanced Data Quality: AI algorithms validate incoming data in real time, reducing errors and ensuring high-quality data, potentially obviating thousands of hours of manual review in a single trial.
- Automated Data Cleaning: AI automates the data review process, significantly cutting down the time spent on manual data cleaning. This not only improves efficiency but also reduces costs.
- Real-time Data Analysis: AI analyzes data as soon as it's entered into the system, providing researchers with immediate insights and facilitating quicker, more informed decision-making.
- Predictive Analytics: AI forecasts trends and outcomes based on existing data, enabling researchers to make proactive adjustments to their clinical trials.
- Improved Patient Recruitment: AI aids in identifying suitable candidates for clinical trials by analyzing patient data, speeding up the recruitment process and increasing the trials' overall efficiency.
The transformative potential of AI is such that it has shifted the roles within data management, transforming data managers into data scientists. As AI automates traditional tasks such as data cleaning and processing, these professionals can now focus on strategic tasks, like participating early in clinical trial strategy development, determining the best data capture methods, and data analysis.
Moreover, AI's influence is visible in the rise of wearable devices and bedside monitors. This revolutionizes clinical trials' execution by:
- Streamlined Data Management: AI effectively manages high volumes of data from various sources, including electronic health records, wearable devices, and lab results, making data management more efficient.
- Enhanced Data Security: AI algorithms help detect and prevent data breaches, ensuring the integrity and confidentiality of sensitive clinical data.
- Accelerated Clinical Trials: AI's ability to automate various processes, from data collection to analysis, can help reduce the duration of clinical trials, getting drugs to market faster.
- Personalized Treatments: AI can analyze individual patient data to develop personalized treatment plans, thereby potentially improving patient outcomes in clinical trials.
These developments underscore AI's role in bringing unprecedented flexibility and adaptability to clinical trials, allowing data scientists to manage a wide range of data types and efficiently process all relevant data during a trial setup or closure. Furthermore, innovative tools leveraging advanced AI conduct data review more efficiently and on a larger scale. These tools bolster data quality and reduce cycle times, enhancing overall clinical data management efficiency and productivity.
3. Challenges and the Road Ahead
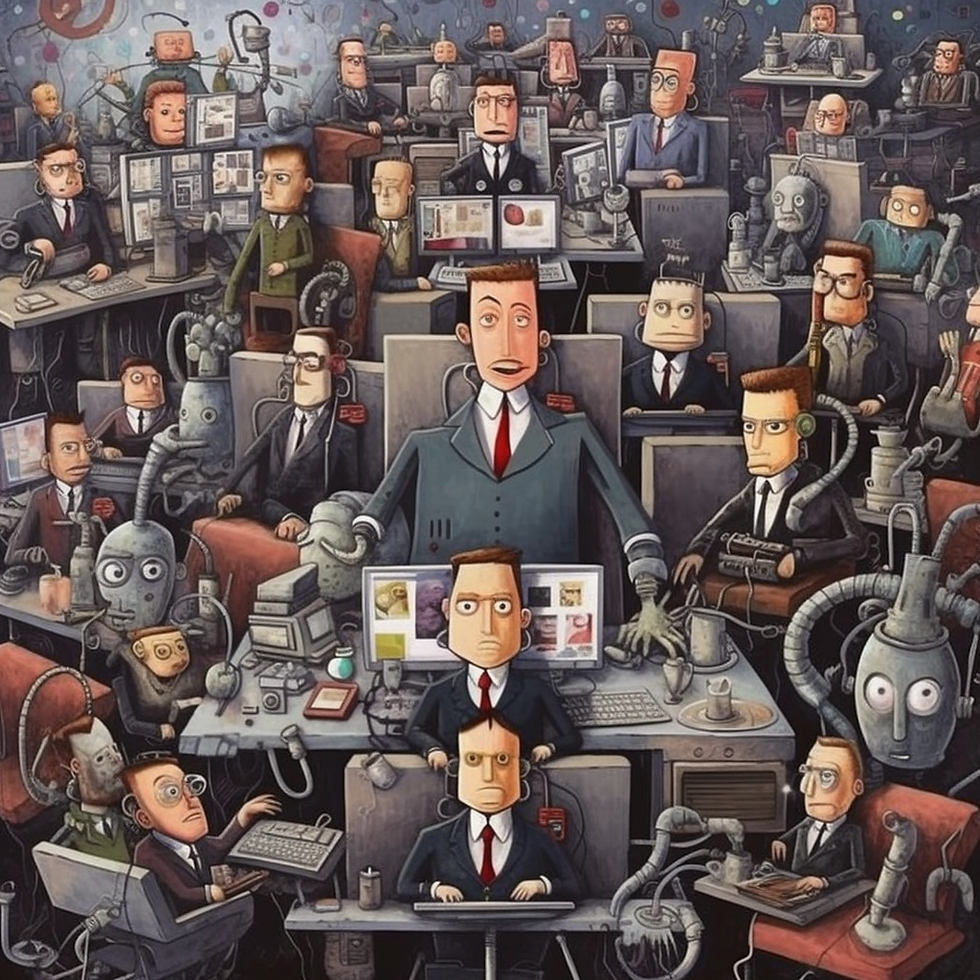
Artificial Intelligence's influence permeates numerous aspects of life sciences, including research, drug discovery, personalized medicine, genomics research, medical imaging, drug repurposing, biomarker discovery, clinical decision support, pharmacovigilance, and even the prediction of future trends. However, careful consideration is needed to address ethical implications such as data privacy, algorithmic bias, transparency, and human-AI collaboration.
While AI's transformative potential is significant, it's important to acknowledge the obstacles clinical data management teams encounter on the road to modernization, like:
- The 'Black Box' Problem: A prominent challenge in the pharmaceutical industry is skepticism towards AI, particularly its 'black box' nature. Explainable AI, which can elucidate its decision-making processes, may be a potential solution to this mistrust.
- Disparate Data Sources: Traditional data management often involves sorting through multiple unstructured data formats. Consolidating structured data in databases becomes crucial to streamline this process.
- Increasing Data Volume: The rapid advancement in technology has led to an exponential increase in data collection. Traditional data collection and analysis practices struggle to keep up with this data deluge. To address this, it's essential to adopt innovative methods like data visualizations and risk-based data cleaning.
- Embracing New Technology: Despite the technological feasibility of fully digital clinical data management, achieving success requires a comprehensive understanding of AI/ML technologies. Clinical data management teams must steer the adoption of these technologies, envisioning the future of data management.
- Data Privacy and Security: The extensive use of AI and machine learning in clinical data management introduces data privacy and security concerns. Organizations need to fortify their security measures to safeguard sensitive clinical data.
AI's transformative influence on clinical data management is diverse, including automated data cleaning, the role evolution of data managers into data scientists, enhanced data collection from diverse sources, and unprecedented levels of flexibility and adaptability in clinical trials. AI-equipped data management platforms, enable data review in a more efficient, scalable manner. These tools rapidly identify anomalous data, enhance data quality, boost productivity, and reduce cycle times.
Conclusion: AI's Promising Role in Clinical Data Management and Beyond
In summary, AI holds immense potential to reshape pharmaceutical systems and clinical data management. The promise of a future with accelerated drug development, improved patient outcomes, and enhanced efficiency is exciting. However, to truly harness AI's power and drive innovation in the sector, it's critical to address the associated challenges. By taking a proactive approach, investing in the right tools and technologies, and promoting continuous education and development of data management teams, we can unlock the full potential of AI in clinical data management and, on a larger scale, the pharmaceutical sector.
As we chart the path forward, investment in AI education and training, along with the establishment of robust governance frameworks, will be key to harnessing AI's full potential while safeguarding patient safety and privacy. With a proactive and informed approach, we can indeed unlock the transformative power of AI in pharmaceutical systems and clinical data management, leading to a brighter, healthier future.
Comments